Get Ready for Robot Revolution: NVIDIA CEO Says AI ‘s Next Frontier is Physical

The world of Artificial Intelligence (AI) is about to take a giant leap from our screens to the real world! This exciting news comes from none other than Jensen Huang, the CEO of NVIDIA, a leading innovator in the field of Artificial Intelligence technology.
AI Goes Physical: Beyond Phones and Desktops
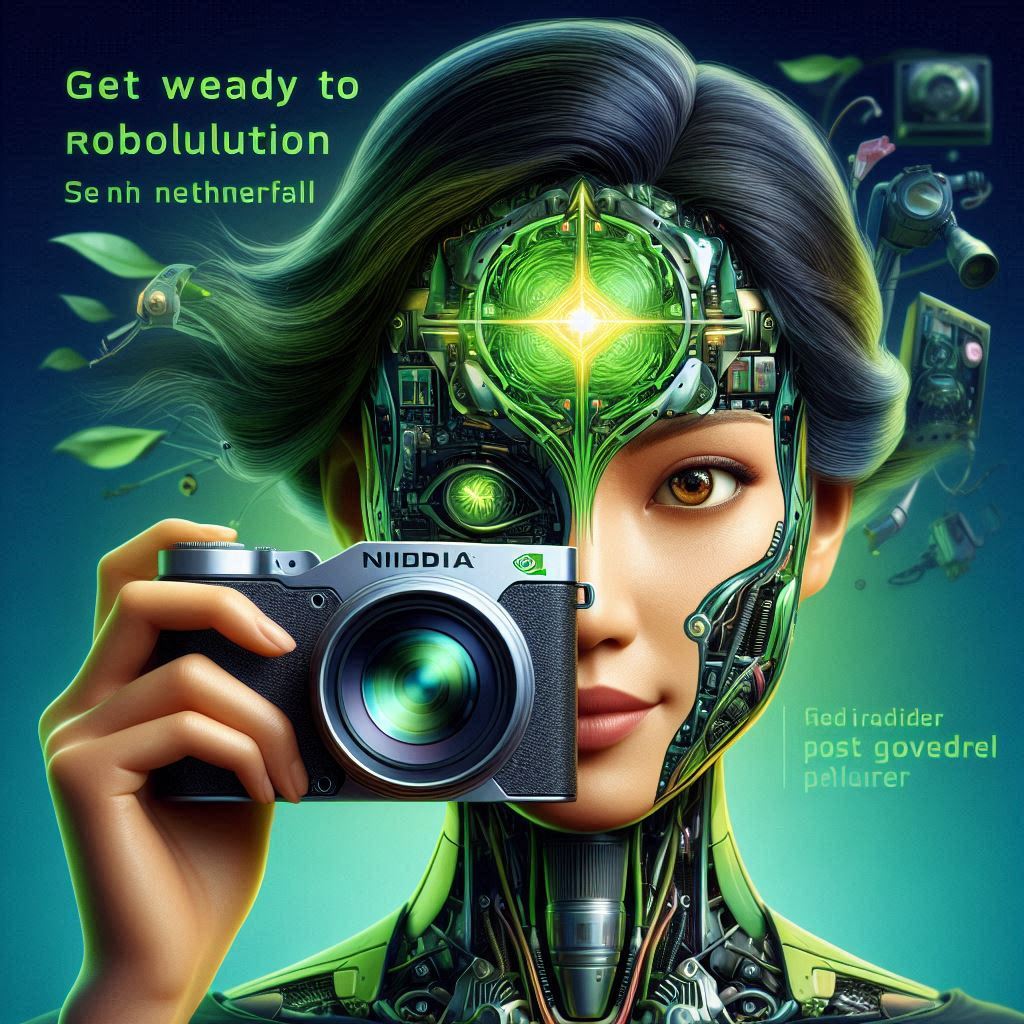
Speaking at COMPUTEX, a major tech event in Taiwan, Huang declared that AI is poised to move beyond the confines of smartphones and desktops. He envisions a future where “AI understands the laws of physics, AI that can work among us.” In simpler terms, we’re on the cusp of a robot revolution!
Robots are Here, and They’re Here to Stay
Huang emphasizes the reality of robotics, stating, “Robotics is here. Physical AI is here. This is not science fiction, and it’s being used all over Taiwan. It’s just really, really exciting.” This isn’t just theoretical; practical applications are already underway.
NVIDIA’s Isaac: Powering the Robot Workforce
At the forefront of this revolution is NVIDIA’s Isaac AI robot development platform. Isaac is already being used in Taiwanese factories and warehouses to streamline operations. It’s also playing a crucial role in the research, development, and production of “tens of millions” of AI-powered robots, including industrial arms and even humanoids.
What Does This Mean for the Future?
Huang’s announcement signifies a significant shift in the trajectory of AI development. Here are some potential implications:
- Enhanced Automation: AI-powered robots can automate various tasks in industries like manufacturing and logistics, leading to increased efficiency and productivity.
- Human-Robot Collaboration: Robots are not here to replace humans, but to work alongside us. Imagine robots handling hazardous or repetitive tasks, freeing humans for more strategic roles.
- Advancements in Robotics: As AI technology evolves, so will our robotic counterparts. We can expect more sophisticated robots with improved dexterity, decision-making capabilities, and adaptability.
The Future is Now
The future of AI is no longer a distant dream. NVIDIA’s advancements with Isaac and Huang’s visionary leadership suggest that physical AI is here to stay. The coming years will likely witness a surge in robotic applications across various sectors, potentially transforming the way we live and work.
What are some Recent Breakthroughs in Physical AI?

Here are some recent breakthroughs in physical AI:
- Generative AI Tools: ChatGPT, developed by OpenAI, reached mass adoption in record time. It reset the course of the industry, allowing users to access powerful neural networks via a web app. Microsoft and Google followed suit, integrating chatbot-based assistants into office software for tasks like summarizing emails, drafting reports, and generating slide decks.
- AI Robot CyberRunner: Researchers at ETH Zurich created an Artificial Intelligence robot named CyberRunner. Its task is to learn how to play the popular labyrinth marble game, demonstrating Artificial Intelligence’s ability to push boundaries in physical skill.
- Alternative Physics Discovery: Columbia University’s AI program observed physical phenomena and uncovered unexpected variables—a necessary step toward developing new physics theories. These variables include energy, mass, and velocity, as seen in Einstein’s iconic equation E=MC².
These breakthroughs highlight Artificial Intelligence rapid progress and its impact on various domains, from generative tools to physical applications! 🌟🤖
Other Companies are working on Physical AI
Several companies are at the forefront of developing physical Artificial Intelligence. Here are some notable ones:
Additionally, there are companies integrating Artificial Intelligence software with hardware for real-world impact:
Remember, these companies contribute to the exciting evolution of physical AI, bridging the gap between theory and practical applications! 🌟🤖
Successful Real-World Applications of Physical AI

Physical Artificial Intelligence  extends beyond digital realms and interacts with the tangible world. Here are some successful real-world applications:
- AI-Based Visual Inspections:
- Climate Change Mitigation:
- Robotics and IoT:
- Physical World Challenges:
- Unlike digital-to-digital AI, physical AI faces messy, less predictable environments. Opening a real-world door, with its diverse handles, remains a complex task for AI systems.
In summary, It bridges the gap between digital data and tangible interactions, transforming how we live and work! 🌟🤖Â
Challenges in Implementing Physical AI
Implementing it presents several challenges:
- Sensor Integration and Perception:
- Artificial Intelligence-powered robots need accurate perception of their environment. Integrating sensors (such as cameras, lidar, and radar) to provide real-time data is crucial. However, handling noisy or incomplete sensor inputs remains a challenge.
- Robustness and Safety:
- Ensuring that Artificial Intelligence systems operate safely in dynamic environments is essential. Robustness against unexpected scenarios (e.g., sudden obstacles) and fail-safe mechanisms are critical.
- Adaptability and Generalization:
- Artificial Intelligence models trained in specific conditions may struggle when faced with novel situations. Achieving adaptability and generalization—especially in unstructured environments—is an ongoing challenge.
- Energy Efficiency:
- They often operate on limited power. Balancing performance with energy consumption is crucial for prolonged operation.
- Dexterity and Manipulation:
- Developing robots with human-like dexterity remains challenging. Precise manipulation of objects requires advanced control algorithms and mechanical design.
- Human-Robot Interaction:
- Designing intuitive interfaces for humans to interact with robots is complex. Natural language understanding, gesture recognition, and social cues play a role.
- Ethical and Legal Considerations:
- As robots become more integrated into daily life, ethical questions arise. Issues like privacy, accountability, and bias need careful attention.
- Cost and Scalability:
- Building them can be expensive. Balancing cost-effectiveness with performance is a challenge, especially for widespread adoption.
In summary, addressing these challenges requires interdisciplinary collaboration, innovation, and ongoing research! 🌟🤖
NVIDIA’s Stock Party Keeps Booming: AI Powerhouse Soars Past $1,000!
